Simple Models of Complex Chaotic Systems
J. C. Sprott
Department of Physics, University
of Wisconsin, Madison, Wisconsin 53706
(Received 28 August 2007; accepted 8 December 2007)
ABSTRACT
Many phenomena in the real world are inherently complex and
involve many dynamical variables interacting nonlinearly
through feedback loops and exhibiting chaos,
self-organization, and pattern formation. It is useful to ask if
there
are generic features of such systems, and if so, how
simple can such systems be and still display these
features. This paper describes several such systems that are accessible
to
undergraduates and might serve as useful examples of complexity.
Ref: J. C. Sprott,
Am. J. Phys.
76, 474-480 (2008)
The complete paper is available in PDF
format.
Return to Sprott's Books and Publications.
Fig. 1. Largest Lypunov exponent for a collection of 472 fully
connected artificial neural networks in Eq. (5) with N = 101 and random Gaussian weights.
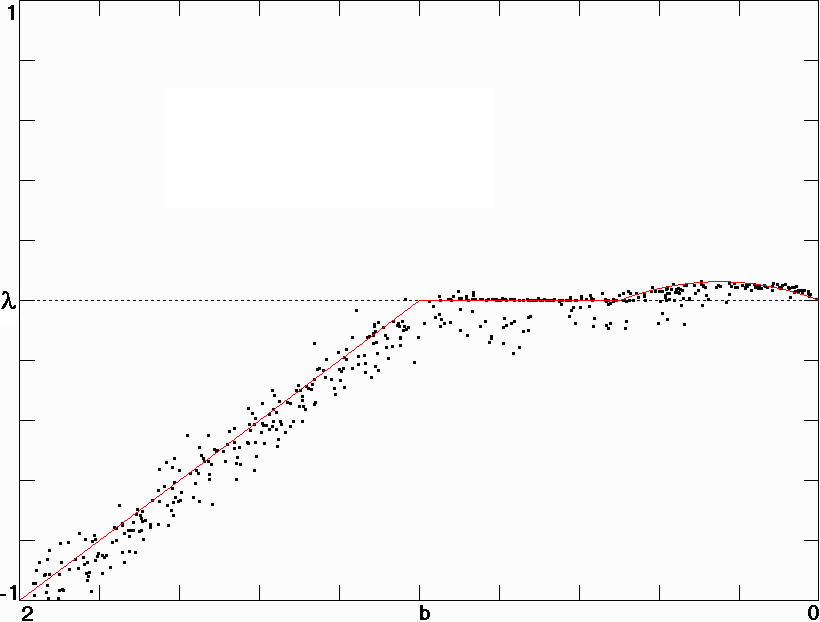
Fig. 2. Largest Lyapunov exponent
for the sparse circulant neural network in Eq. (7) with N = 101 and four-neighbor
interactions.
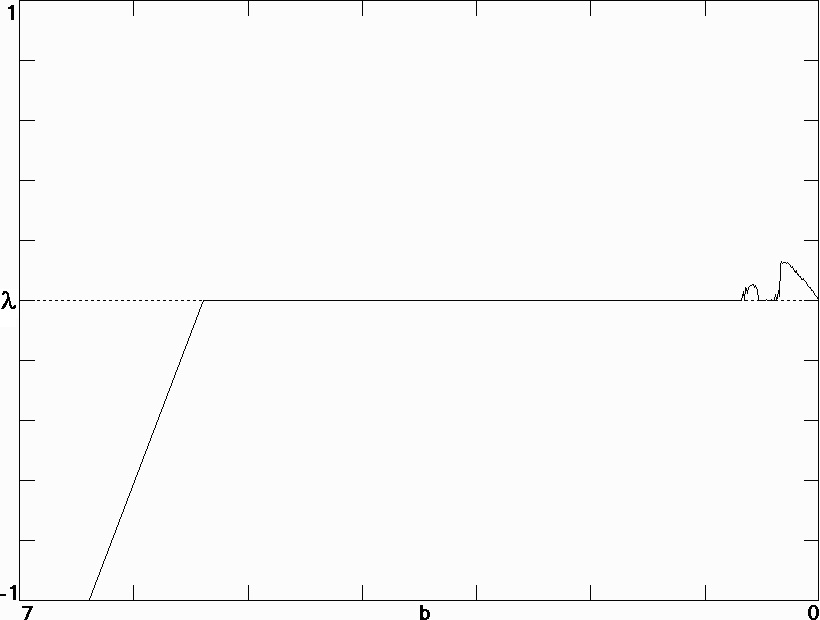
Fig. 3. Spatiotemporal plot of
chaos in a sparse circulant neural network with N = 101 and b = 0.25.
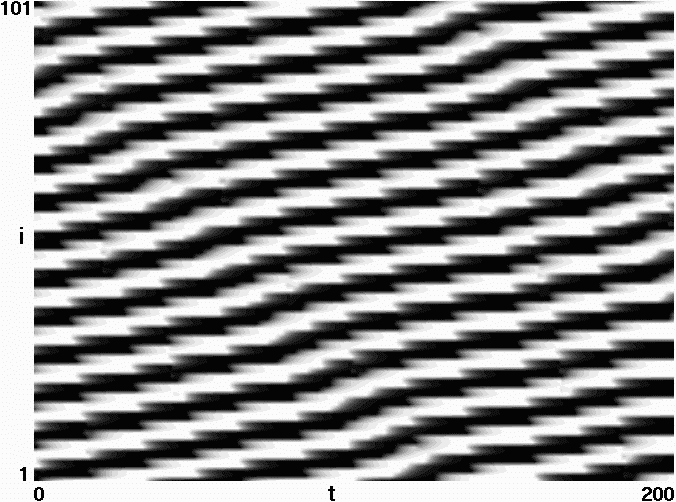
Fig. 4. Largest Lyapunov exponent
for the hyperlabyrinth system in Eq. (8) with N = 101.
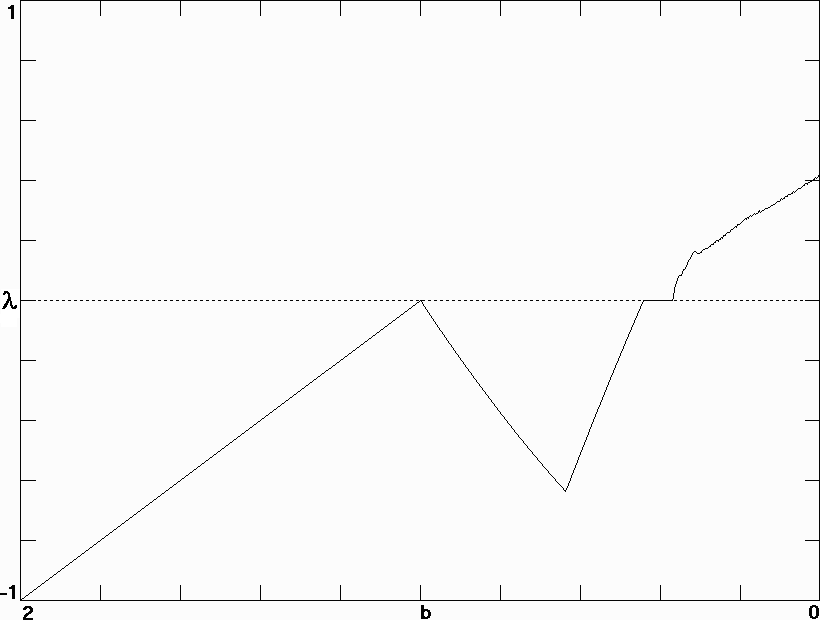
Fig. 5. Spatiotemporal plot of
hyperlabyrinth chaos for N =
101 and b = 0.25.
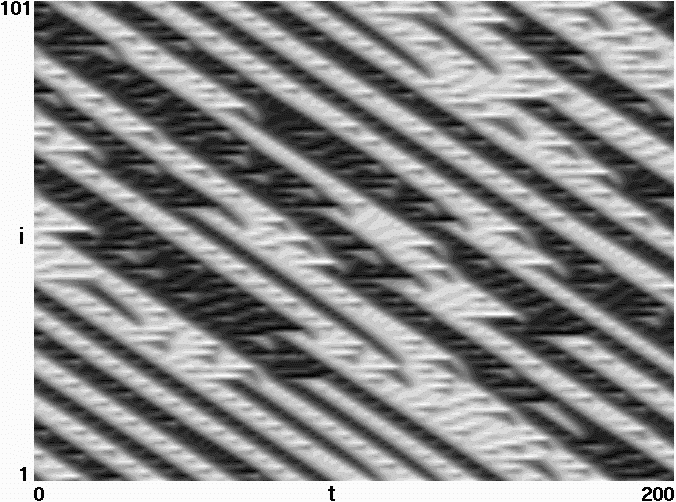
Fig. 6. Largest Lyapunov exponent
for the Lotka-Volterra model in Eq. (9) with N = 101.
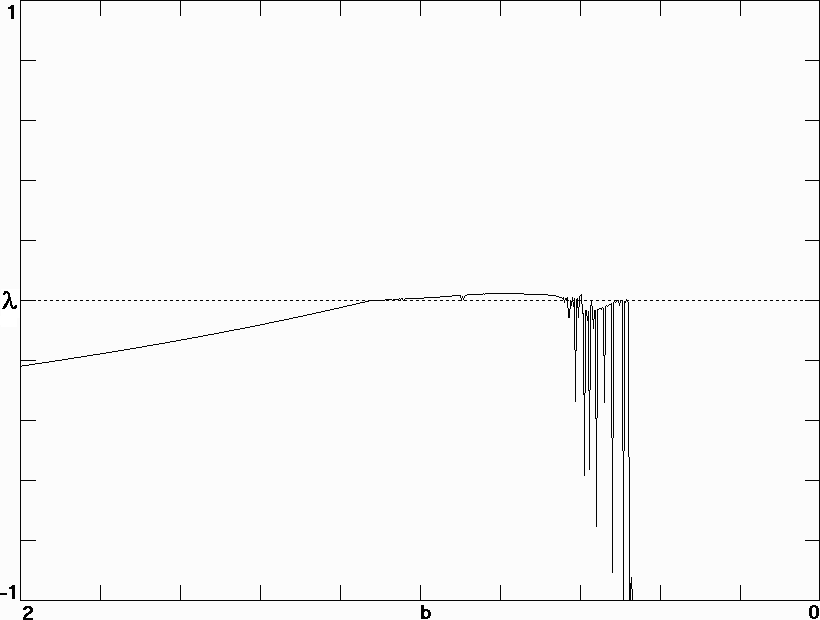
Fig. 7. Spatiotemporal plot of
the Lotka-Volterra model for N
= 101 and b = 0.8.
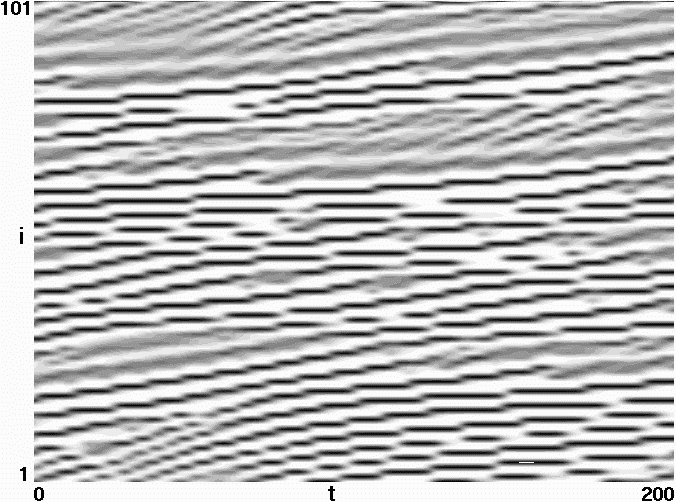
Fig. 8. Largest Lyapunov exponent
for the delayed differential equation model in Eq. (10) with f(x)
=
sin x and N = 100.
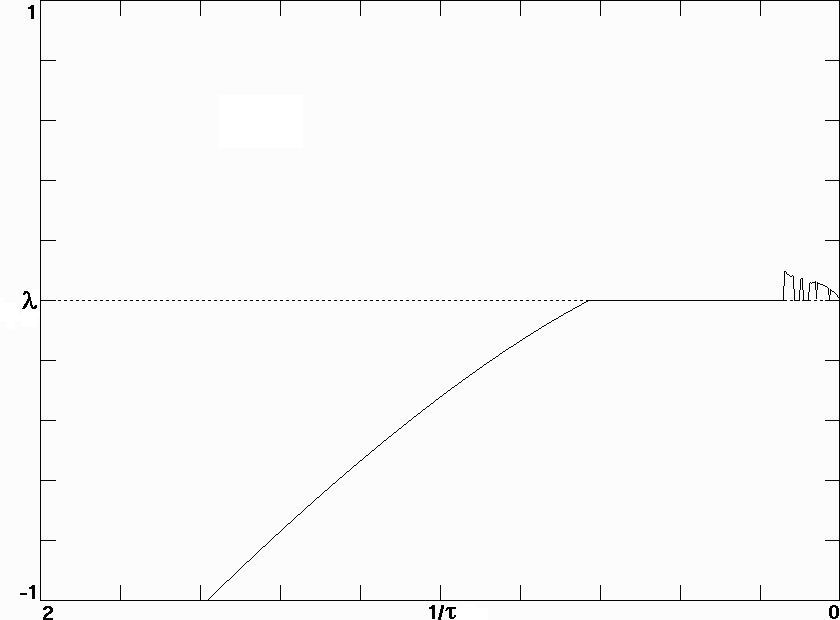
Fig. 9. Strange attractor for the
delayed differ4ential equation model in Eq. (10) with f(x)
=
sin x, tau = 8 and N = 100.
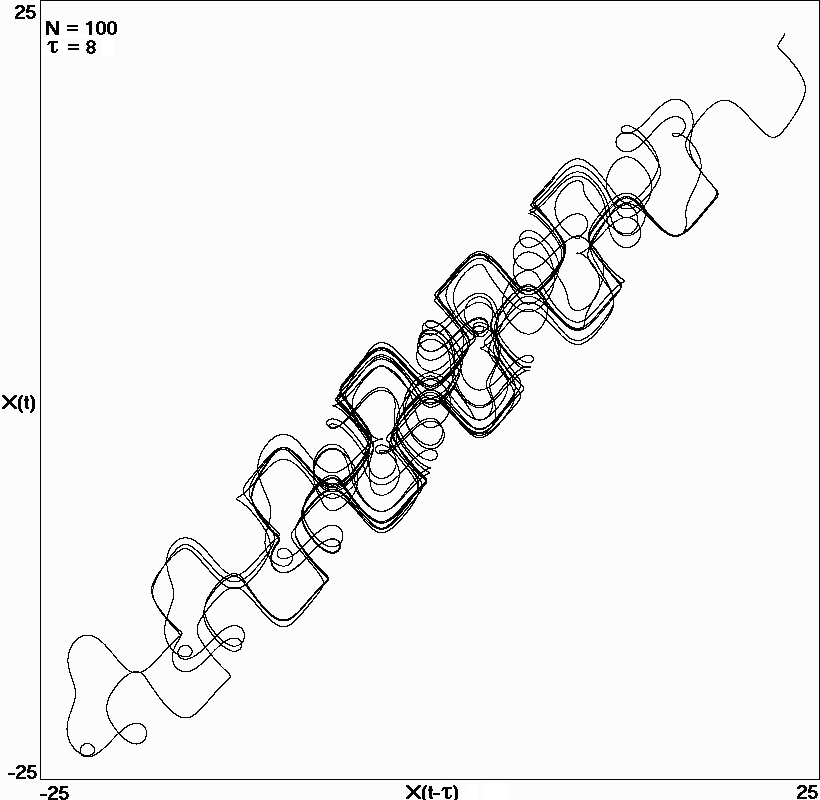
Fig. 10. Largest Lyapunov
exponent for the PDE model in Eq. (13) with N = 101.
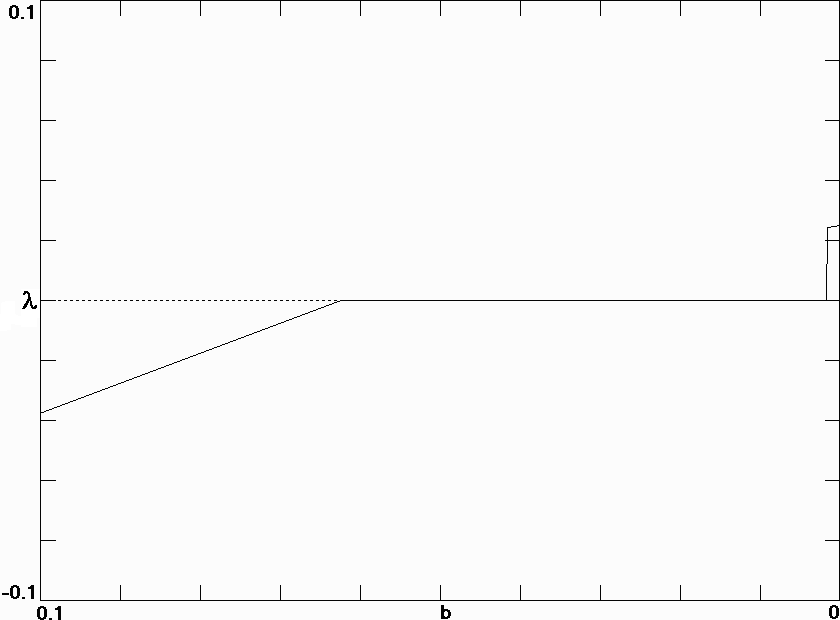
Fig. 11. Spatiotemporal plot of
the PDE model in Eq. (12) for N
= 101 and b = 0.
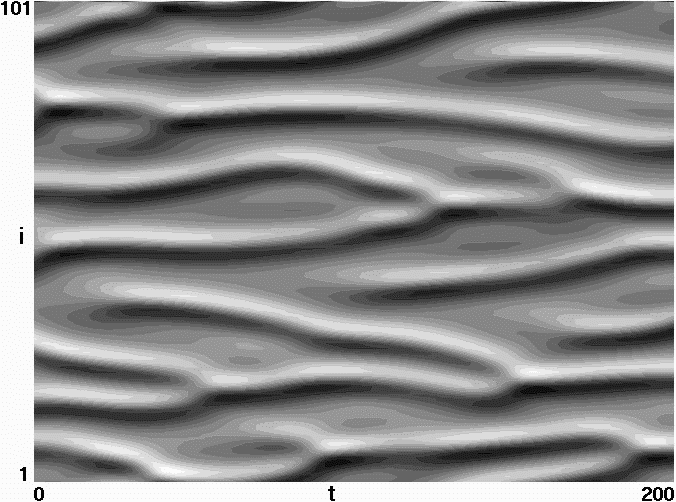